Guest post originally published on the Gemini Open Cloud blog by Patrick Fu, CEO of Gemini Open Cloud
The GPU computation is asynchronous to the POD itself. Typically, the process running on the POD copies data to the GPU memory and issues a CUDA instruction to the GPU to execute the calculation (known as GPU kernel). When the GPU kernel finishes with the computation, it will issue a sync request to wake up the POD and copies the computation results back to the main memory.
GPU Kernels are non-preemptable and cannot be interrupted. Therefore, even after GPU partitioning, the actual amount of GPU usage by each POD is still unpredictable, which may still lead to underutilization, or performance delays. For this reason, we need to implement collaboration between the scheduler front-end, a device manager, and a scheduler backend to achieve fair share scheduling.
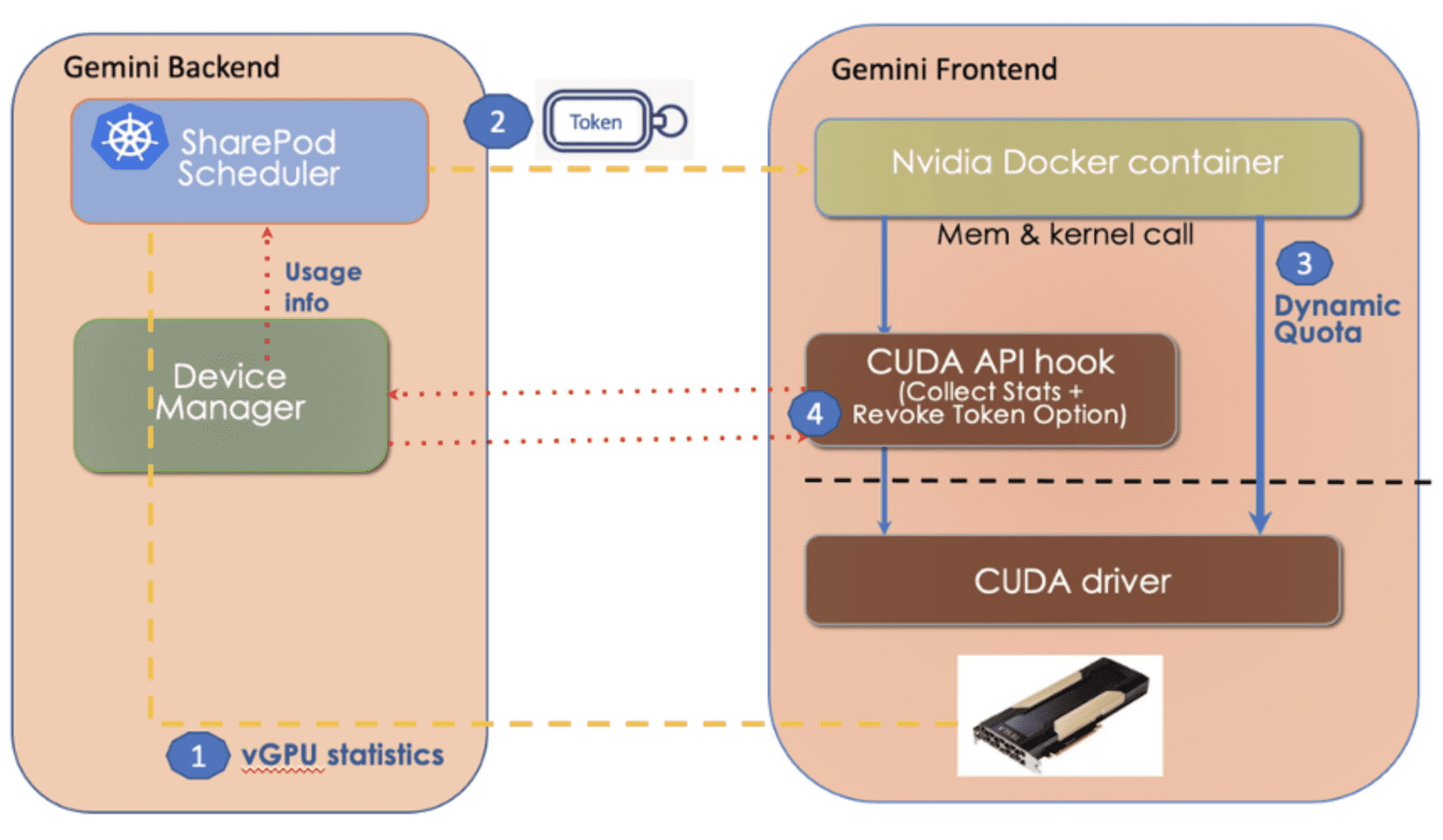
Figure 5 shows how the Gemini scheduler achieves fair share scheduling for ML workload. It consists of an event-driven monitoring subsystem (#1) to collect the GPU utilization for the Device Manager. The Gemini scheduler will calculate on a real-time basis the next POD that should be scheduled. There are 2 pieces of information the scheduler needs to calculate:
- The POD that’s currently furthest away from its target GPU % utilization.
- The amount of time this POD should be given to run on the target GPU.
This information is encoded in a token and dispatched to the target worker node (#2 & #3). As these processes reiterates, the PODs should be getting closer to its target GPU quota. In the case a POD exceeded its quota, the token will be revoked (#4) and the POD will not be eligible to be scheduled.
We will briefly explain each of these 3 subsystems:
Event driven Monitoring
- As mentioned above, GPU kernels are not preemptable. In order to capture and measure runtime kernel execution behavior without introducing synchronization points between the CPU and GPU, our event driven monitoring subsystem piggybacks the SYNC event issued by the GPU kernel to record the amount of GPU time used by a sharePOD and stores the utilization statistics with the backend device manager.
- The goal of the monitor is to identify kernel bursts from applications, and correctly record their actual start time and end time for execution
Token-based time-sharing scheduler
- Once a sharePOD’s GPU kernel is completed, the physical GPU becomes available. Our backend must then schedule the next sharePOD to run on the worker node of the corresponding GPU.
- We implemented a dynamic quota strategy based on the estimated kernel burst time to adapt to dynamic workload patterns. Our approach is to let the API hook provide some statistics of the kernel burst of its client to the scheduler. Then the scheduler uses a smooth function to gradually adjust the token quota of a client according to its estimated burst time from the client
- The target physical GPU and the dynamic quota is embedded in a token and dispatched to the corresponding worker node. Thus, the token serves as a mechanism for the front-end load distribution and fair sharing of GPU resources by the sharePODs.
Token Revocation
- To minimize context switch and interruptions, we allow each sharePOD to execute multiple GPU kernels. However, because GPU kernels are non-preemptable, we want to prevent runaway sharePODs. The token revocation scheme is designed to avoid non-preemptive kernels from exceeding their scheduling time quota. A token becomes invalid when its quota is expired, and its hook library must request a new token from the scheduler for future kernel execution.
Summary
In summary, we have explained how we customize the default Kube scheduler to allow a physical GPU to be shared by multiple POD’s and how we collect their GPU utilization to dynamically adjust the time slice we allocate to the PODs running ML workload.
K8s Scheduler Series Reference
- Kubernetes worker nodes
- Kube scheduler Framework
- Creating a Kube schedule plugin
- Sample Scheduler framework Plugins
- Gang Scheduling
- Capacity Scheduling
- GPU Binpacking
Gemini Open Cloud is a CNCF member and a CNCF-certified Kubernetes service provider. With more than ten years of experience in cloud technology, Gemini Open Cloud is an early leader in cloud technology in Taiwan.